Topic two sample chi square: The two sample chi-square test is a crucial statistical method used to determine if there is a significant difference between two categorical variables. This test helps researchers analyze relationships and make informed decisions based on data. In this article, we will explore the fundamentals, applications, and step-by-step procedures for conducting a two sample chi-square test effectively.
Table of Content
- Search Results for "two sample chi square"
- Introduction to Two Sample Chi-Square Tests
- Understanding Chi-Square Tests
- Types of Chi-Square Tests
- Steps to Perform a Chi-Square Test
- Assumptions and Limitations
- Assumptions and Conditions
- Step-by-Step Guide to Performing a Two Sample Chi-Square Test
- Reporting the Results
- Practical Applications of Two Sample Chi-Square Tests
- Common Mistakes and How to Avoid Them
- Advanced Topics
- Conclusion
- YOUTUBE: Video hướng dẫn về kiểm định Chi-Square, giải thích cách thực hiện và ý nghĩa của kiểm định này trong các tình huống thực tế.
Search Results for "two sample chi square"
Here are the synthesized search results for the keyword "two sample chi square":
-
Definition and Use
The two sample chi square test is a statistical method used to determine if there is a significant association between two categorical variables. It compares the observed frequencies of the variables with the frequencies that would be expected if there were no association.
-
Statistical Analysis
This test is commonly employed in research fields such as social sciences, medicine, and biology to analyze data sets where researchers want to assess the relationship between two variables.
-
Application in Research
Researchers often use the two sample chi square test to determine whether there is a significant difference between observed and expected frequencies in their data, allowing them to draw conclusions about the relationship between variables.
-
Software and Tools
Various statistical software packages such as SPSS, R, and SAS provide tools to perform the two sample chi square test, making it accessible for researchers and analysts in various fields.
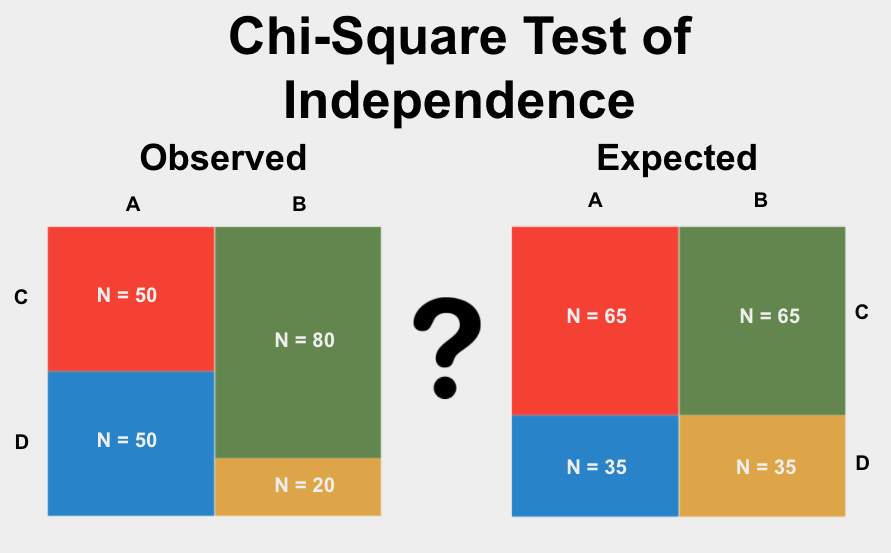
READ MORE:
Introduction to Two Sample Chi-Square Tests
The Two Sample Chi-Square Test is a statistical method used to determine whether there is a significant difference between the distributions of two independent samples. It compares observed frequencies to expected frequencies under the null hypothesis that the two samples come from the same distribution. This test is applicable in various fields, including biology, marketing, and social sciences.
The test statistic for the Two Sample Chi-Square Test is calculated as follows:
\[
\chi^2 = \sum \frac{(O_i - E_i)^2}{E_i}
\]
where \( O_i \) represents the observed frequencies and \( E_i \) represents the expected frequencies.
Here are the steps to perform a Two Sample Chi-Square Test:
- Formulate the null hypothesis (\(H_0\)) and alternative hypothesis (\(H_a\)):
- \(H_0\): The two samples come from the same distribution.
- \(H_a\): The two samples come from different distributions.
- Calculate the observed frequencies for each category in both samples.
- Calculate the expected frequencies for each category assuming the null hypothesis is true.
- Compute the chi-square statistic using the formula given above.
- Determine the degrees of freedom (\(df\)):
- For equal sample sizes: \(df = k - 1\)
- For unequal sample sizes: \(df = k - 2\)
- Compare the chi-square statistic to the critical value from the chi-square distribution table at a chosen significance level (\(\alpha\)).
- Make a decision:
- If the chi-square statistic is greater than the critical value, reject \(H_0\).
- If the chi-square statistic is less than or equal to the critical value, do not reject \(H_0\).
For example, imagine a researcher wants to determine if two different teaching methods result in different levels of student performance. By collecting performance data from students taught using each method, calculating observed and expected frequencies, and performing the Two Sample Chi-Square Test, the researcher can infer whether the teaching methods have a statistically significant impact on performance.
Understanding Chi-Square Tests
The Chi-Square test is a statistical method used to determine if there is a significant association between categorical variables. It compares the observed frequencies in a dataset to the expected frequencies, assuming no association between variables. The test helps to ascertain whether any differences between the observed and expected frequencies are due to chance or some underlying relationship.
Chi-Square tests are commonly used in hypothesis testing to analyze categorical data. The two most common types of Chi-Square tests are the Chi-Square Test of Independence and the Chi-Square Goodness of Fit Test. Below is a detailed explanation of these tests and how they are conducted.
Types of Chi-Square Tests
- Chi-Square Test of Independence: This test determines if there is an association between two categorical variables. For example, it can be used to examine whether gender is related to voting preference.
- Chi-Square Goodness of Fit Test: This test assesses how well the observed data fit an expected distribution. For instance, it can check if a die is fair by comparing the observed frequency of each face with the expected frequency.
Steps to Perform a Chi-Square Test
-
Define the Hypotheses:
- Null Hypothesis (\(H_0\)): Assumes no association between variables or that the observed distribution fits the expected distribution.
- Alternative Hypothesis (\(H_A\)): Assumes an association between variables or that the observed distribution does not fit the expected distribution.
- Choose the Significance Level: Typically set at 0.05, this is the threshold for determining statistical significance.
- Collect and Organize Data: Gather the data into a contingency table or frequency table.
- Calculate the Expected Frequencies: For each cell in the table, calculate the expected frequency using the formula: \[ E_i = \frac{(row \ total \times column \ total)}{grand \ total} \]
- Compute the Chi-Square Statistic: Use the formula: \[ \chi^2 = \sum \frac{(O_i - E_i)^2}{E_i} \] where \(O_i\) is the observed frequency and \(E_i\) is the expected frequency.
- Determine the Degrees of Freedom: The degrees of freedom for the test is calculated as: \[ df = (number \ of \ rows - 1) \times (number \ of \ columns - 1) \]
- Compare to the Critical Value: Compare the calculated Chi-Square statistic to the critical value from the Chi-Square distribution table. If the statistic exceeds the critical value, reject the null hypothesis.
- Draw Conclusions: Interpret the results in the context of the research question, considering both statistical and practical significance.
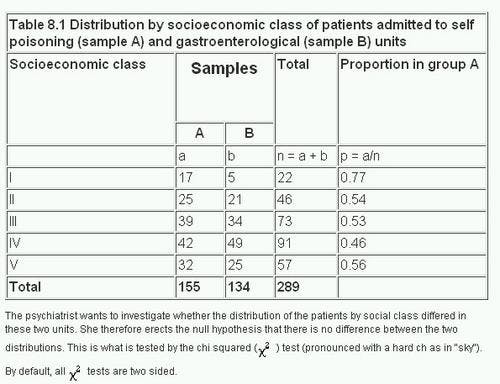
Assumptions and Limitations
- Data should be randomly sampled and observations must be independent.
- The test is suitable for categorical data; using it for continuous data requires appropriate categorization.
- Expected frequencies should be at least 5 to ensure the validity of the test results.
The Chi-Square test is a powerful tool in statistical analysis, helping to reveal associations between variables and the goodness of fit of observed data to expected distributions. Understanding its application and limitations ensures accurate and meaningful results in your data analysis.
Assumptions and Conditions
The chi-square test of independence is a statistical method used to determine if there is a significant association between two categorical variables. To ensure the validity of this test, several assumptions and conditions must be met:
- Random Sampling: The data should be collected through a process of random sampling to ensure that every member of the population has an equal chance of being included in the sample.
- Independence of Observations: Each observation should be independent of the others. This means that the outcome of one observation does not influence the outcome of another.
- Categorical Data: The variables being analyzed must be categorical. This means they should represent distinct groups or categories.
- Expected Cell Frequency: The expected frequency for each cell in the contingency table should be at least 5. This helps ensure the reliability of the chi-square approximation.
Below is a summary of the key elements required for the chi-square test of independence:
Key Element | Description |
Test Definition | A non-parametric test used to determine if there is a significant association between two categorical variables. |
Test Purpose | To check for a significant difference between the observed and expected frequencies in categorical data. |
Null Hypothesis | There is no association between the two variables. |
Assumptions | Random selection, independence of observations, categorical variables, and expected cell frequency of at least 5. |
It is important to handle these assumptions carefully. Violating these assumptions can lead to inaccurate conclusions. For example, if the observations are not independent, the chi-square test may indicate a false association between the variables.
Additionally, the chi-square test does not provide information about the strength or direction of the association. To measure the strength of the association, additional statistics such as Cramer's V or Phi can be used.
In conclusion, by adhering to these assumptions and conditions, the chi-square test of independence can be a powerful tool for analyzing relationships between categorical variables.
Step-by-Step Guide to Performing a Two Sample Chi-Square Test
Performing a two sample chi-square test involves several key steps. This guide will walk you through the process, ensuring you can confidently apply this statistical method.
1. Defining Hypotheses
Begin by establishing the null and alternative hypotheses:
- Null Hypothesis (H0): The two samples come from the same distribution.
- Alternative Hypothesis (Ha): The two samples come from different distributions.
2. Selecting Significance Level
Choose a significance level (\(\alpha\)), commonly set at 0.05, which defines the probability of rejecting the null hypothesis when it is actually true.
3. Calculating Observed and Expected Frequencies
Organize your data into a contingency table and calculate the observed frequencies. Next, compute the expected frequencies using the formula:
\[ E = \frac{( \text{row total} \times \text{column total} )}{\text{grand total}} \]
4. Computing the Chi-Square Statistic
Use the observed and expected frequencies to calculate the chi-square statistic (\(\chi^2\)):
\[ \chi^2 = \sum \frac{(O - E)^2}{E} \]
where \(O\) represents the observed frequency and \(E\) represents the expected frequency.
5. Determining Degrees of Freedom
The degrees of freedom (df) for the test is calculated as:
\[ \text{df} = (r - 1) \times (c - 1) \]
where \(r\) is the number of rows and \(c\) is the number of columns in the contingency table.
6. Comparing to Critical Value
Find the critical value from the chi-square distribution table for the chosen significance level and the calculated degrees of freedom. Compare the calculated chi-square statistic to the critical value:
- If \(\chi^2\) > critical value, reject the null hypothesis.
- If \(\chi^2\) ≤ critical value, fail to reject the null hypothesis.
7. Interpreting the Results
Based on the comparison, draw a conclusion about whether there is a significant difference between the distributions of the two samples. If you reject the null hypothesis, it suggests the samples come from different distributions.
Example
Category | Sample 1 | Sample 2 |
---|---|---|
A | 40 | 50 |
B | 30 | 20 |
C | 20 | 30 |
Calculate the expected frequencies and then use the formula to find the chi-square statistic. Determine the degrees of freedom and compare the statistic to the critical value to conclude the test.
Reporting the Results
When reporting the results of a two sample chi-square test, it is crucial to present the information clearly and concisely. Follow these steps to ensure that your report is thorough and understandable:
1. Restate the Hypotheses
Begin by clearly restating your null and alternative hypotheses. This helps to remind the reader of the purpose of the test and what you were investigating.
Example: The null hypothesis (\(H_0\)) states that there is no significant association between the two categorical variables. The alternative hypothesis (\(H_A\)) states that there is a significant association between the two variables.
2. Describe the Test Performed
Provide a brief description of the chi-square test performed, including the type of test (e.g., chi-square test of independence or homogeneity) and the reason for choosing this test.
Example: A chi-square test of independence was performed to examine the relationship between gender and voting preference.
3. Report the Chi-Square Statistic
Report the calculated chi-square statistic, degrees of freedom, and the sample size. Also, include the p-value to indicate whether the result is statistically significant.
- Use the format:
\(\chi^2\) (df, N = sample size) = chi-square value, p = p-value
- Ensure to present the chi-square value and p-value to two decimal places.
Example: The chi-square test revealed a significant association between gender and voting preference, \(\chi^2\) (1, N = 100) = 6.76, p = 0.009.
4. Interpret the Results
Interpret the results in the context of your study. Explain what the significant or non-significant result means in practical terms.
Example: This result suggests that gender is significantly associated with voting preference, with males more likely to prefer candidate A compared to females.
5. Present the Crosstabulation Table
Include a crosstabulation table to show the observed and expected frequencies for each combination of categories. This helps to visualize the data and supports the statistical findings.
Category | Observed Frequency | Expected Frequency |
---|---|---|
Category 1 | O1 | E1 |
Category 2 | O2 | E2 |
6. Discuss the Implications
Finally, discuss the implications of your findings. Consider how the results contribute to the broader field of study and any potential limitations.
Example: These findings highlight the importance of considering gender in voting behavior studies. However, the study is limited by its sample size and the specific population surveyed, which may affect the generalizability of the results.

Practical Applications of Two Sample Chi-Square Tests
The two-sample chi-square test is a powerful statistical tool used in various fields to analyze categorical data and determine if there is a significant association between two variables. Here are some practical applications:
Market Research
In market research, companies use two-sample chi-square tests to compare consumer preferences between two different groups. For instance, a company may want to test if there is a significant difference in the preference for a new product between urban and rural consumers.
- Example: A survey is conducted to compare the preference for a new beverage between 500 urban and 500 rural respondents. The data collected can be analyzed using a chi-square test to determine if location influences consumer preference.
Healthcare Studies
Healthcare researchers use two-sample chi-square tests to compare the effectiveness of different treatments or interventions. This helps in determining if there is a significant difference in outcomes between two groups of patients.
- Example: A clinical trial comparing the effectiveness of two treatments for hypertension. The results are categorized into patients who showed improvement and those who did not for both treatment groups. A chi-square test can then be used to analyze the data.
Educational Assessments
In education, two-sample chi-square tests can be applied to compare the performance or behaviors of students from different educational programs or schools. This helps in evaluating the impact of different teaching methods or curricula.
- Example: Comparing the graduation rates of students from two different high schools. The data on whether students graduated or not from each school can be analyzed using a chi-square test to determine if there is a significant difference in graduation rates.
These applications demonstrate the versatility of the two-sample chi-square test in analyzing categorical data across various fields, making it an essential tool for researchers and analysts.
Common Mistakes and How to Avoid Them
When conducting a two-sample Chi-Square test, it's crucial to avoid common mistakes that can compromise the validity of your results. Below are some typical errors and tips on how to prevent them:
Incorrect Binning of Data
One of the most frequent mistakes is the incorrect binning of continuous data into categorical data. Ensure that:
- Data is binned in a way that each category has a sufficient number of observations.
- Categories are mutually exclusive and exhaustive.
- Binning should be based on logical and statistical rationale to maintain the integrity of the analysis.
Misinterpretation of Results
A significant Chi-Square result indicates an association but not causation. To avoid misinterpretation:
- Understand that the test only shows whether there is a statistically significant relationship between variables.
- Consider the context and other underlying factors that might influence the results.
- Use additional statistical tests or measures, like Cramer's V or Phi coefficient, to determine the strength of the association.
Ignoring Assumptions
Chi-Square tests have several assumptions that need to be met:
- Expected frequency in each cell of the contingency table should be 5 or more. If this condition is violated, use Fisher's exact test instead.
- Data should be collected through random sampling to avoid biases.
- Observations should be independent of each other.
Small Sample Sizes
Small sample sizes can lead to unreliable results:
- Ensure the sample size is large enough to provide reliable expected frequencies.
- Consider combining categories with low frequencies or using exact tests for small samples.
Errors in Calculation
Errors in calculating the Chi-Square statistic can occur if not careful:
- Double-check the observed and expected frequencies before performing calculations.
- Use statistical software to reduce manual calculation errors.
- Ensure proper computation of degrees of freedom, which depends on the number of categories.
Not Reporting Effect Size
Reporting only p-values without effect size can be misleading:
- Include effect size measures like Cramer's V or Phi coefficient to provide insight into the strength of the association.
- Effect size helps in understanding the practical significance of the results, especially in large sample sizes where even small differences can be statistically significant.
By being aware of these common mistakes and taking steps to avoid them, you can ensure that your Chi-Square test results are both accurate and meaningful.
Advanced Topics
This section delves into more sophisticated aspects of the two-sample Chi-Square test, exploring alternative statistical methods, power analysis, and software tools that enhance the application and interpretation of Chi-Square tests.
Alternative Statistical Tests
In some cases, alternative statistical tests may be more appropriate than the Chi-Square test. Here are a few alternatives:
Kolmogorov-Smirnov Two Sample Test
The Kolmogorov-Smirnov test is a non-parametric test used to compare the distributions of two independent samples. It assesses whether the samples come from the same distribution without assuming a specific distribution for the data.
- Advantages: It is useful for small sample sizes and does not assume a normal distribution.
- Disadvantages: It is less powerful than parametric tests when the assumptions of parametric tests are met.
Quantile-Quantile Plot
A Quantile-Quantile (Q-Q) plot is a graphical tool to compare two probability distributions by plotting their quantiles against each other. If the distributions are similar, the points will approximately lie on the line \( y = x \).
- Advantages: It provides a visual assessment of the similarity between distributions.
- Disadvantages: It is not a formal test and does not provide a p-value.
Power Analysis and Sample Size Determination
Power analysis is crucial for determining the sample size required to detect an effect of a given size with a certain level of confidence. In the context of Chi-Square tests, the power of the test depends on the effect size, sample size, and significance level.
- Effect Size: Measured by Cramer's V, which indicates the strength of association between variables.
- Sample Size: Larger samples provide more power to detect smaller effects.
- Significance Level: Commonly set at 0.05, it represents the probability of rejecting the null hypothesis when it is true.
Software for Performing Chi-Square Tests
Several software tools can perform Chi-Square tests efficiently. Here are two popular options:
Using Dataplot
Dataplot is a public domain statistical software package that can perform a wide range of statistical analyses, including Chi-Square tests. It is user-friendly and provides comprehensive documentation for conducting various tests.
- Installation: Dataplot can be downloaded from the official website.
- Usage: Users can input their data and select the Chi-Square test function to perform the analysis.
Using JMP
JMP is a statistical software suite by SAS that offers extensive capabilities for data analysis, including Chi-Square tests. It provides an intuitive interface and robust features for data visualization and statistical modeling.
- Installation: JMP requires a license and can be installed from the SAS website.
- Usage: JMP offers easy-to-use menus and commands for performing Chi-Square tests, with detailed output and graphical representations of results.
By exploring these advanced topics, researchers can enhance their understanding and application of Chi-Square tests, ensuring more accurate and insightful data analysis.
Conclusion
The two-sample chi-square test is a robust statistical tool used to determine if there are significant differences between two categorical datasets. By comparing observed frequencies to expected frequencies under the null hypothesis, this test helps to identify whether any observed deviations are likely due to chance or indicative of a significant association.
Throughout this guide, we have explored various aspects of performing a two-sample chi-square test, from understanding the test's purpose and assumptions to interpreting and reporting the results. Key takeaways include:
- The importance of defining clear hypotheses and ensuring that the data meets the necessary assumptions for the chi-square test.
- A step-by-step approach to calculating the chi-square statistic and determining its significance.
- Common mistakes to avoid, such as incorrect data binning and misinterpretation of results.
- Advanced topics, including alternative tests and software options for conducting chi-square tests.
By carefully following the outlined procedures and best practices, researchers can effectively utilize the two-sample chi-square test to draw meaningful conclusions from categorical data. Whether in market research, healthcare, education, or other fields, the insights gained from this test can guide informed decision-making and contribute to a deeper understanding of the studied phenomena.
As with any statistical method, it's essential to complement chi-square tests with other analyses and contextual knowledge to ensure robust and reliable conclusions. Continuous learning and application of these methods will enhance the accuracy and relevance of your statistical findings.
In summary, the two-sample chi-square test is a valuable method for examining the relationship between categorical variables. By adhering to proper methodologies and being mindful of potential pitfalls, researchers can leverage this test to uncover significant patterns and relationships in their data.
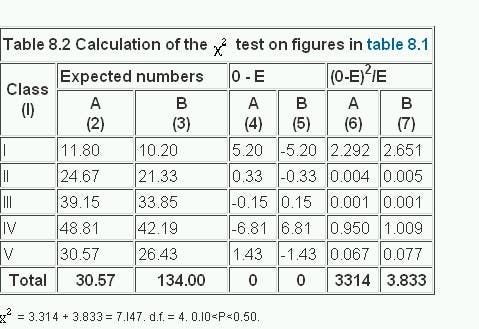
Video hướng dẫn về kiểm định Chi-Square, giải thích cách thực hiện và ý nghĩa của kiểm định này trong các tình huống thực tế.
Kiểm Định Chi-Square
READ MORE:
Video giải thích đơn giản về kiểm định Chi-Square, hướng dẫn cách thực hiện và ý nghĩa của kiểm định này trong các tình huống thực tế.
Kiểm Định Chi-Square [Giải Thích Đơn Giản]