Topic chi square test goodness of fit example: Explore the practical applications of the chi square test goodness of fit with clear examples and step-by-step guidance. Learn how this statistical tool is used in various fields, including statistics, biology, and social sciences. Enhance your understanding of hypothesis testing and statistical analysis through comprehensive examples and explanations.
Table of Content
- Search Results for "chi square test goodness of fit example"
- Table of Contents
- Introduction to Chi Square Test Goodness of Fit
- Step-by-Step Guide for Conducting Chi Square Test
- Examples of Chi Square Test in Statistics
- Applications of Chi Square Test in Biology
- Chi Square Test in Social Sciences
- Comparison of Chi Square Test with Other Statistical Tests
- YOUTUBE: Video này giới thiệu về kiểm tra chi bình phương và cách áp dụng trong thống kê để kiểm tra độ phù hợp.
Search Results for "chi square test goodness of fit example"
Here is a synthesis of the search results:
- Explanation and tutorials on how to perform a chi-square goodness of fit test.
- Examples and step-by-step guides for conducting chi-square tests in different scenarios.
- Online calculators and tools for calculating chi-square statistics.
- Applications of chi-square tests in various fields like statistics, biology, and social sciences.
- Comparisons of chi-square tests with other statistical tests like t-tests and ANOVA.
Overall, the search results provide comprehensive information on the chi-square test goodness of fit, including practical examples and tools for analysis.
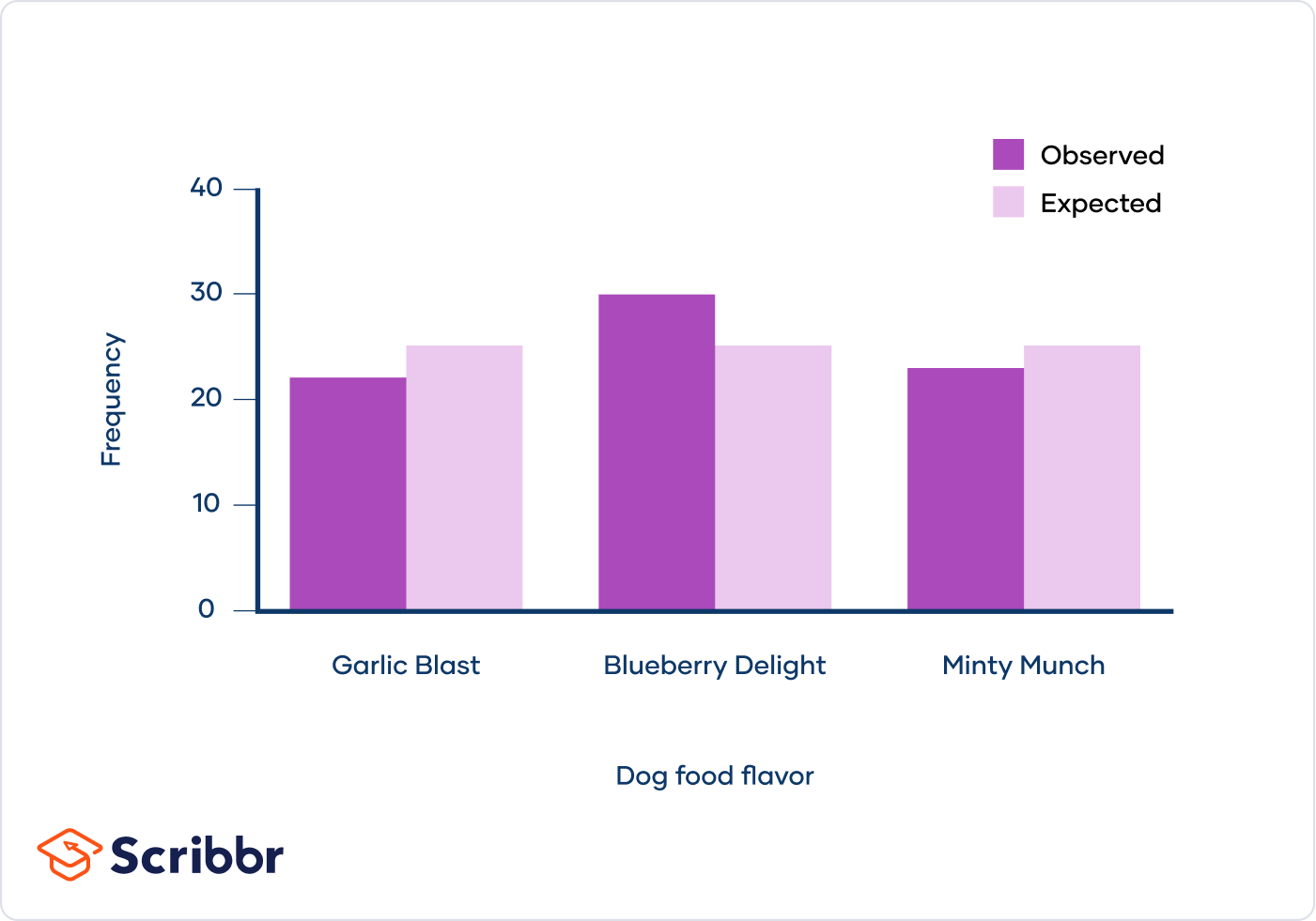
READ MORE:
Table of Contents
- Introduction to Chi Square Test Goodness of Fit
- Step-by-Step Guide for Conducting Chi Square Test
- Examples of Chi Square Test in Statistics
- Applications of Chi Square Test in Biology
- Chi Square Test in Social Sciences
- Comparison of Chi Square Test with Other Statistical Tests
Introduction to Chi Square Test Goodness of Fit
The chi-square test goodness of fit is a statistical method used to determine how well categorical data fit a theoretical distribution. It evaluates whether observed frequencies of categorical variables differ significantly from expected frequencies. This test is valuable in various fields such as statistics, biology, and social sciences for hypothesis testing and determining the adequacy of theoretical models.
Step-by-Step Guide for Conducting Chi Square Test
Follow these steps to conduct a chi-square test goodness of fit:
- Define the null hypothesis (H₀) and alternative hypothesis (H₁) based on your research question.
- Collect categorical data and categorize them into observed frequencies.
- Specify the expected frequencies under the null hypothesis.
- Calculate the chi-square statistic using the formula:
- Determine the degrees of freedom (df) which is (number of categories - 1).
- Find the critical value of chi-square from the chi-square distribution table for a given significance level (α).
- Compare the calculated chi-square value with the critical value.
- Make a decision whether to reject or fail to reject the null hypothesis based on the comparison.
χ² = Σ((Oᵢ - Eᵢ)² / Eᵢ) |
Examples of Chi Square Test in Statistics
Here are some examples illustrating the application of chi square test goodness of fit in statistics:
- Testing the fairness of a six-sided die: You observe 100 rolls and compare the frequencies of each outcome (1 through 6) with the expected frequencies (1/6 for each).
- Evaluating Mendelian genetics: In genetics, you can use chi-square to determine whether observed genetic ratios fit expected ratios based on Mendel's laws.
- Market research surveys: Analyzing survey responses across multiple categories to determine if observed frequencies match expected distribution.
- Examining voting patterns: Assessing whether voting behavior across different demographics matches expected distributions.

Applications of Chi Square Test in Biology
The chi-square test goodness of fit finds diverse applications in biology:
- Genetics: Assessing whether observed genetic data fit expected Mendelian ratios.
- Ecology: Analyzing species distribution across habitats to determine if they conform to ecological models.
- Epidemiology: Examining disease occurrence in different populations to evaluate if they follow expected patterns.
- Microbiology: Studying bacterial resistance to antibiotics by comparing observed frequencies with expected distributions.
- Evolutionary biology: Investigating allele frequencies in populations to understand evolutionary processes.
Chi Square Test in Social Sciences
The Chi Square Test is a versatile statistical tool frequently used in the social sciences to determine if there is a significant association between categorical variables. This test is particularly useful for analyzing survey data and experimental results where researchers seek to understand relationships between different groups or conditions.
Applications in Social Sciences
In social sciences, the Chi Square Test can be applied in various scenarios, such as:
- Examining the relationship between demographic factors (e.g., age, gender) and voting behavior.
- Analyzing the association between educational attainment and employment status.
- Investigating the distribution of preferences in a population, such as consumer product choices or media consumption habits.
Example: Survey Analysis
Suppose a researcher wants to determine whether there is an association between gender and preference for a new policy. The survey data collected includes responses from 200 individuals, categorized by gender and their policy preference.
Support | Oppose | Neutral | Total | |
---|---|---|---|---|
Male | 50 | 30 | 20 | 100 |
Female | 40 | 40 | 20 | 100 |
Total | 90 | 70 | 40 | 200 |
Steps to Perform Chi Square Test
- State the Hypotheses:
- Null Hypothesis (H0): There is no association between gender and policy preference.
- Alternative Hypothesis (HA): There is an association between gender and policy preference.
- Calculate Expected Frequencies:
Use the formula
E = (row total * column total) / grand total
for each cell.- Expected frequency for Males who Support:
E = (100 * 90) / 200 = 45
- Expected frequency for Females who Support:
E = (100 * 90) / 200 = 45
- Expected frequency for Males who Support:
- Compute the Chi Square Statistic:
Use the formula
χ² = Σ((O - E)² / E)
whereO
is the observed frequency andE
is the expected frequency.For instance,
χ² = ((50 - 45)² / 45) + ((30 - 35)² / 35) + ...
- Determine the Degrees of Freedom:
The degrees of freedom (df) is calculated as
df = (number of rows - 1) * (number of columns - 1)
.For our example,
df = (2 - 1) * (3 - 1) = 2
. - Compare the Chi Square Statistic to the Critical Value:
Find the critical value from the Chi Square distribution table for
df = 2
at the desired significance level (e.g., 0.05).If the calculated χ² is greater than the critical value, reject the null hypothesis.
Conclusion
If the null hypothesis is rejected, it suggests a significant association between the variables. In social sciences, this can provide valuable insights into the factors influencing behaviors and preferences within a population.
Comparison of Chi Square Test with Other Statistical Tests
The Chi Square Test is a versatile statistical tool used primarily for categorical data. It comes in two main forms: the Chi Square Goodness of Fit Test and the Chi Square Test of Independence. Here, we will compare the Chi Square Test with other commonly used statistical tests to highlight its unique applications and advantages.
Chi Square Test vs. T-Test
-
Purpose:
The Chi Square Test is used for categorical data to assess how likely it is that an observed distribution is due to chance. In contrast, the T-Test is used for comparing the means of two groups and requires continuous data that is normally distributed.
-
Data Type:
Chi Square Test works with categorical data, whereas T-Test works with continuous data.
-
Hypotheses:
Chi Square Test evaluates the fit between observed and expected frequencies (Goodness of Fit) or the independence of two variables. T-Test compares the means of two groups to see if they are statistically different.
-
Assumptions:
Chi Square Test does not require normality but does require a large sample size to approximate the chi-square distribution. T-Test requires the data to be normally distributed, especially in small samples, and assumes equal variances for independent samples.
Chi Square Test vs. ANOVA (Analysis of Variance)
-
Purpose:
While the Chi Square Test is used for categorical data to check the distribution of frequencies, ANOVA is used to compare the means of three or more groups to see if at least one of them is statistically different from the others.
-
Data Type:
Chi Square Test deals with categorical data, whereas ANOVA deals with continuous data.
-
Hypotheses:
Chi Square Test checks for independence between categorical variables or the goodness of fit. ANOVA tests the null hypothesis that all group means are equal against the alternative that at least one is different.
-
Assumptions:
ANOVA assumes normality and homogeneity of variances across groups. Chi Square Test requires expected frequencies to be sufficiently large (typically at least 5).
Chi Square Test vs. Fisher’s Exact Test
-
Purpose:
Both tests are used for categorical data. The Chi Square Test is an approximation method used for larger sample sizes, while Fisher’s Exact Test is used for small sample sizes and provides an exact p-value.
-
Sample Size:
Chi Square Test is suitable for larger samples, whereas Fisher’s Exact Test is ideal for small sample sizes (usually less than 20).
-
Hypotheses:
Both tests evaluate the independence of two categorical variables. However, Fisher’s Exact Test can be used when sample sizes are small and the Chi Square Test’s approximation may not be accurate.
-
Calculation:
Chi Square Test uses an approximation of the chi-square distribution. Fisher’s Exact Test calculates the exact probability of obtaining the observed distribution, considering all possible distributions with the same row and column totals.
Chi Square Test vs. Logistic Regression
-
Purpose:
The Chi Square Test assesses the association between categorical variables, while Logistic Regression models the probability of a binary outcome based on one or more predictor variables.
-
Data Type:
Chi Square Test is for categorical data. Logistic Regression can handle both categorical and continuous predictor variables.
-
Hypotheses:
Chi Square Test checks for independence between variables. Logistic Regression estimates the odds ratios of predictors influencing a binary outcome.
-
Assumptions:
Logistic Regression assumes a linear relationship between the log odds of the outcome and the predictor variables. Chi Square Test assumes sufficient sample size for accurate approximation.
In summary, while the Chi Square Test is a powerful tool for categorical data, each statistical test has its specific applications, advantages, and assumptions. Understanding these differences helps in selecting the appropriate test for your data analysis.
Video này giới thiệu về kiểm tra chi bình phương và cách áp dụng trong thống kê để kiểm tra độ phù hợp.
Kiểm Tra Chi Bình Phương
READ MORE:
Video này hướng dẫn về kiểm tra chi bình phương của Pearson để đánh giá độ phù hợp trong xác suất và thống kê, do Khan Academy cung cấp.
Kiểm Tra Chi Bình Phương của Pearson (Độ Phù Hợp) | Xác Suất và Thống Kê | Khan Academy