Topic example of chi square test for goodness of fit: Explore an illustrative example of the Chi Square test for goodness of fit in this article. Learn how this statistical method assesses the variance between expected and observed data, crucial for various research and analytical applications.
Table of Content
- Example of Chi Square Test for Goodness of Fit
- Introduction to Chi Square Test for Goodness of Fit
- Understanding the Chi Square Statistic
- Assumptions of the Chi Square Test
- Steps to Perform a Chi Square Test
- Interpreting Chi Square Test Results
- Example Applications of Chi Square Test for Goodness of Fit
- Comparison with Other Statistical Tests
- YOUTUBE: Xem video về Kiểm định Chi-square cho Phù hợp với các Ví dụ về Sự phù hợp
Example of Chi Square Test for Goodness of Fit
The chi-square test for goodness of fit is a statistical test used to determine if a sample data matches a population. This test compares the observed data to the expected data and checks for discrepancies.
Hypotheses
The hypotheses for the chi-square goodness of fit test are:
- Null hypothesis (H0): The sample data fits the expected distribution.
- Alternative hypothesis (H1): The sample data does not fit the expected distribution.
Example: M&M's Color Distribution
Consider a standard package of milk chocolate M&Ms, which come in six different colors. We want to test if the colors are equally distributed in the package.
Data Collection
We randomly sample 600 M&Ms and observe the following counts for each color:
Color | Observed Count | Expected Count |
Blue | 212 | 100 |
Orange | 147 | 100 |
Green | 103 | 100 |
Red | 50 | 100 |
Yellow | 46 | 100 |
Brown | 42 | 100 |
Calculations
We calculate the chi-square statistic using the formula:
\( \chi^2 = \sum \frac{(O_i - E_i)^2}{E_i} \)
Where \( O_i \) is the observed frequency and \( E_i \) is the expected frequency for each category.
- For Blue: \( \frac{(212 - 100)^2}{100} = 125.44 \)
- For Orange: \( \frac{(147 - 100)^2}{100} = 22.09 \)
- For Green: \( \frac{(103 - 100)^2}{100} = 0.09 \)
- For Red: \( \frac{(50 - 100)^2}{100} = 25 \)
- For Yellow: \( \frac{(46 - 100)^2}{100} = 29.16 \)
- For Brown: \( \frac{(42 - 100)^2}{100} = 33.64 \)
Total chi-square statistic: \( 125.44 + 22.09 + 0.09 + 25 + 29.16 + 33.64 = 235.42 \)
Conclusion
We compare the calculated chi-square statistic to the critical value from the chi-square distribution table with the appropriate degrees of freedom (number of categories - 1 = 6 - 1 = 5). If the calculated value is greater than the critical value, we reject the null hypothesis.
In this example, the high chi-square value indicates that the observed distribution of M&M colors does not fit the expected distribution, suggesting that the colors are not equally distributed.
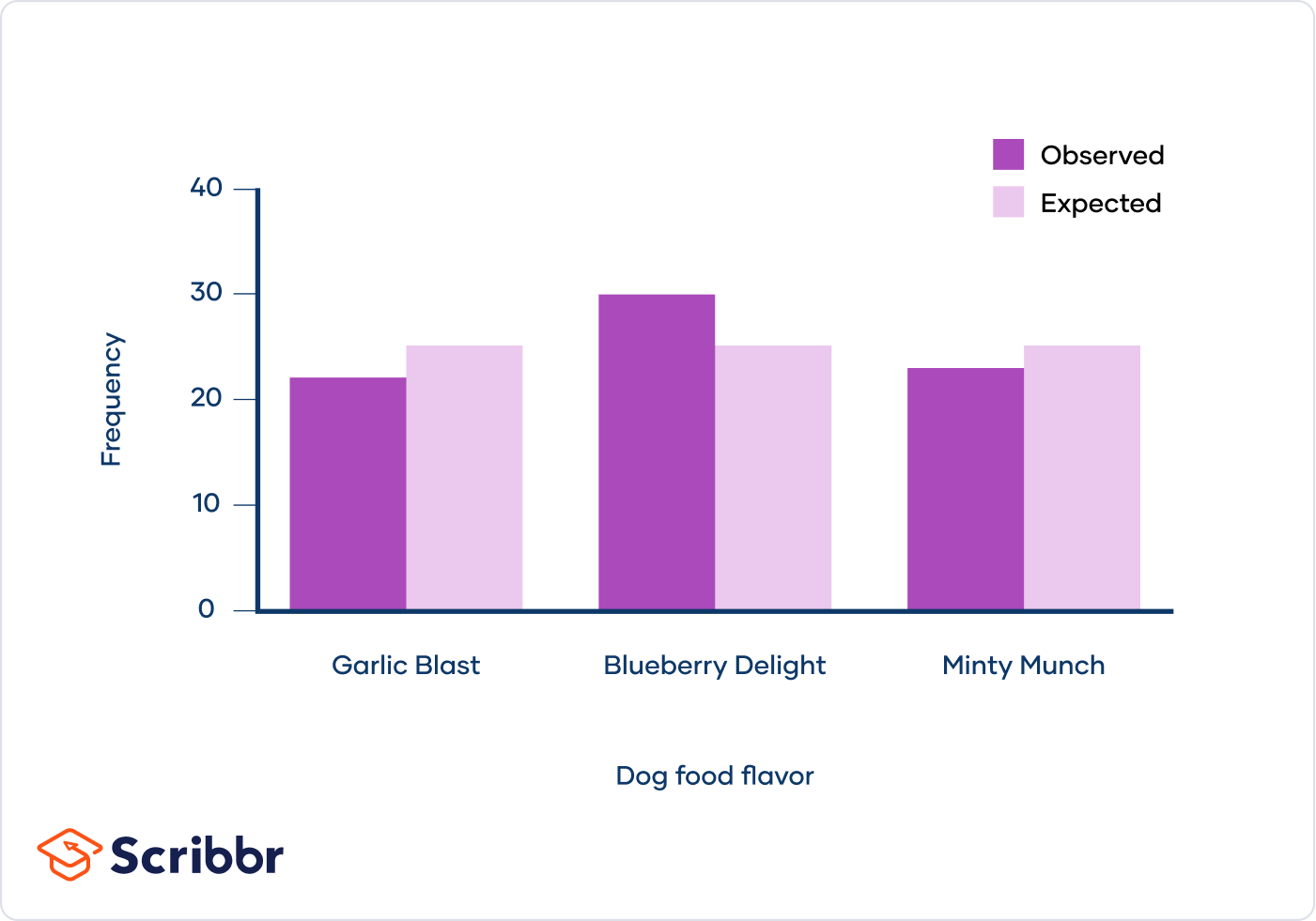
READ MORE:
Introduction to Chi Square Test for Goodness of Fit
The Chi Square Test for Goodness of Fit is a statistical test used to determine whether a set of categorical data fits a specific theoretical distribution. It's particularly useful when we want to assess how well an observed frequency distribution matches an expected frequency distribution.
Key applications of this test include:
- Evaluating whether observed data (such as survey results, categorical counts, etc.) follow a hypothesized distribution (like a uniform distribution, normal distribution, etc.).
- Comparing observed frequencies with expected frequencies to assess the goodness of fit.
- Testing theoretical models against real-world data to validate assumptions or theories.
The test relies on the Chi Square statistic, which measures the difference between observed and expected frequencies, normalized by expected frequencies. A higher Chi Square value indicates a greater discrepancy between observed and expected data, suggesting a poor fit to the hypothesized distribution.
Assumptions of the Chi Square Test for Goodness of Fit typically include:
- Independence of observations (each observation should be independent of others).
- Appropriateness of the theoretical distribution being tested (e.g., normal distribution, uniform distribution).
- Sufficient sample size to ensure reliable results.
To perform the test, one typically follows these steps:
- Formulate the null hypothesis and alternative hypothesis regarding the distribution.
- Collect observed data and classify them into categories.
- Determine expected frequencies under the null hypothesis.
- Calculate the Chi Square statistic based on observed and expected frequencies.
- Compare the calculated Chi Square value with critical values from the Chi Square distribution table or use a statistical software to determine the p-value.
- Interpret the results: If the p-value is less than the chosen significance level (commonly 0.05), reject the null hypothesis and conclude that there is significant evidence that the observed data does not fit the expected distribution.
This test is widely used in various fields including biology, economics, social sciences, and more, where categorical data analysis is essential for drawing conclusions and making decisions based on empirical evidence.
Understanding the Chi Square Statistic
The Chi Square statistic is a measure used in statistical tests to determine the extent of discrepancy between observed and expected frequencies in categorical data. It quantifies how well the observed data fit a theoretical distribution.
Key points to understand about the Chi Square statistic include:
- Calculation: It is calculated as the sum of the squared differences between observed (O) and expected (E) frequencies, divided by the expected frequencies for all categories.
- Formula: \( \chi^2 = \sum \frac{(O_i - E_i)^2}{E_i} \), where \( O_i \) is the observed frequency and \( E_i \) is the expected frequency for each category.
- Degrees of Freedom: Degrees of freedom in the Chi Square test depend on the number of categories and are crucial in determining critical values from the Chi Square distribution table for hypothesis testing.
- Interpretation: A higher Chi Square value indicates a greater discrepancy between observed and expected frequencies, suggesting a poorer fit to the theoretical distribution. Conversely, a lower value suggests a better fit.
- Application: The Chi Square statistic is widely used in various fields such as biology, psychology, social sciences, and quality control to test goodness of fit, independence of variables in contingency tables, and homogeneity of proportions among different groups.
Understanding this statistic is fundamental in interpreting the results of Chi Square tests and drawing conclusions based on categorical data analysis.
Assumptions of the Chi Square Test
The Chi Square Test for Goodness of Fit relies on several assumptions to ensure the validity of its results:
- Independence: The observations used in the test must be independent of each other. This means that the occurrence of one event should not influence the occurrence of another event.
- Sample Size: The sample size should be sufficiently large so that expected frequencies are reasonably accurate. In general, each expected frequency should be at least 5 for the Chi Square test to be valid.
- Expected Frequencies: The expected frequencies should be greater than zero for each category under consideration. This ensures that the theoretical distribution being tested against is meaningful.
These assumptions ensure that the Chi Square test produces reliable results and accurately assesses the goodness of fit between observed and expected data.
Steps to Perform a Chi Square Test
- Formulate Hypotheses: Define the null hypothesis \( H_0 \) and alternative hypothesis \( H_a \) regarding the distribution of the categorical data.
- Collect Data: Gather observed data and organize them into categories or groups.
- Calculate Expected Frequencies: Based on the null hypothesis, compute the expected frequencies for each category.
- Compute Chi Square Statistic: Calculate the Chi Square statistic using the formula \( \chi^2 = \sum \frac{(O_i - E_i)^2}{E_i} \), where \( O_i \) is the observed frequency and \( E_i \) is the expected frequency for each category.
- Determine Degrees of Freedom: Calculate the degrees of freedom, which depend on the number of categories involved.
- Compare with Critical Value: Refer to the Chi Square distribution table or use statistical software to find the critical Chi Square value corresponding to your chosen significance level (often 0.05).
- Interpret Results: Compare the calculated Chi Square value with the critical value. If \( \chi^2 \) exceeds the critical value, reject the null hypothesis \( H_0 \), indicating that the observed data do not fit the expected distribution.
- Conclusion: Based on the interpretation, draw conclusions regarding the goodness of fit of the observed data to the hypothesized distribution.
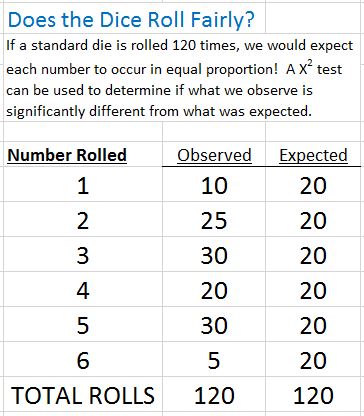
Interpreting Chi Square Test Results
Interpreting Chi Square test results involves several key steps:
- Compare Chi Square Value: Calculate the Chi Square statistic \( \chi^2 \) from your data.
- Find Critical Value: Determine the critical Chi Square value from the Chi Square distribution table corresponding to your chosen significance level (e.g., 0.05).
- Compare with Critical Value: If \( \chi^2 \) is greater than the critical value, reject the null hypothesis \( H_0 \). This indicates that there is significant evidence that the observed data do not fit the expected distribution.
- Consider Degrees of Freedom: Degrees of freedom affect the critical value and must be considered in the interpretation.
- Calculate p-value: Alternatively, calculate the p-value associated with \( \chi^2 \) using statistical software. A p-value less than the chosen significance level (e.g., 0.05) also leads to rejecting \( H_0 \).
- Conclusion: Based on the interpretation of \( \chi^2 \) and the critical value or p-value, conclude whether the observed data fit the expected distribution or not.
It's important to interpret Chi Square test results carefully to draw valid conclusions about the goodness of fit of categorical data to a theoretical distribution.
Example Applications of Chi Square Test for Goodness of Fit
The Chi Square Test for Goodness of Fit finds various applications across different fields:
- Biology: It is used to assess whether observed genetic ratios fit expected Mendelian ratios.
- Market Research: Researchers use it to analyze consumer preferences and determine if they match expected demographic distributions.
- Quality Control: Manufacturers apply it to test whether products conform to specified standards or specifications.
- Education: Educators use it to evaluate exam scores against expected grade distributions.
- Social Sciences: Sociologists employ it to study voting patterns or survey responses across different demographics.
- Environmental Studies: Researchers use it to analyze species distribution data against ecological models.
These examples illustrate the versatility of the Chi Square Test for Goodness of Fit in analyzing categorical data across various disciplines.
Comparison with Other Statistical Tests
The Chi Square Test for Goodness of Fit differs from other statistical tests in several ways:
- T-Test and ANOVA: These tests are used for continuous data analysis, whereas Chi Square is used for categorical data analysis.
- Chi Square Test of Independence: While similar in name, the Chi Square Test for Goodness of Fit assesses how well observed data fit a specific distribution, whereas the Chi Square Test of Independence examines the association between two categorical variables.
- Logistic Regression: Logistic regression is used when the dependent variable is binary, whereas Chi Square tests are used for categorical data with more than two categories.
- Validity: Chi Square tests are robust when assumptions like independence and expected frequencies are met, whereas other tests may require different assumptions for validity.
- Application Scope: Chi Square tests are particularly useful in fields where categorical data analysis is essential, such as biology, social sciences, and market research, while other tests may be more applicable in different contexts.
Understanding these distinctions helps researchers choose the appropriate statistical test based on their data type and research question.
Xem video về Kiểm định Chi-square cho Phù hợp với các Ví dụ về Sự phù hợp
Video Chi-Square Test for Goodness of Fit | Hành vi Thống kê và Ứng dụng
READ MORE:
Xem video về Kiểm định Chi Square và cách áp dụng trong các ví dụ về sự phù hợp với chi-square test.
Video Kiểm định Chi Square | Giải thích và Ứng dụng